FEATURE
No Data, No Problem: How to Get Started with AI
No Data, No Problem: How to Get Started with AI
By Hannah Melia, Head of Marketing, Citrine Informatics, Redwood City, California
As manufacturers move to implement AI in their processes, a few simple tips will help them get started in their search for an AI provider.
A global adhesives manufacturer removed PFAS from an in-market pressure sensitive adhesive in 40% of the planned development time; identifying a breakthrough candidate in just four months. The process is outlined in this case study.
Some adhesives and sealants companies, and those that sell raw materials to them, have started using AI to great effect. Others are more hesitant. Some are worried that they don’t have enough data in the right format to start thinking about using AI. Others are worried that they don’t have the right skill set in-house to get started. This article will outline what value adhesives and sealants companies are already gaining from AI and give practical tips on how to get started and what to look for in an AI provider.
Return on Investment
Dealing with problem ingredients
Companies are swapping out ingredients, such as certain biocides and PFAS chemicals, from product portfolios. This is where scalable AI comes into its own, both in terms of ensuring consistency across a range as well as speed in identifying substitute raw materials. Some AI providers that concentrate on the chemicals and materials space have developed technology to “featurize” chemicals, essentially, converting the molecular structure and chemical formulas into extra data, such as molecular weight or number of hydrogen bonds. By understanding the fingerprint of the problem ingredient and the role it performs in the original formulation, AI can be used to suggest alternatives.
Leading adhesives and sealants companies are already using AI in this way, giving them a competitive advantage. AI will soon become an everyday tool for formulators, and those that are late to the party will find it difficult to catch up.
“AI lets us solve problems with less work. It’s like having a flashlight in a dark room.”
Your team will benefit from understanding these connections more deeply. If you can show that an ingredient has no effect on the target output properties, that is really useful information. Sometimes unintuitive connections will be made, and by reviewing the features that the AI model finds important you will find out something new. Product experts can also use the model to run “what if” scenarios at the computer, before going to the lab and testing promising candidates. By reducing the cost of experimentation, product experts can be more creative and discover completely novel formulations.
“We understand our own laboratory more than before. It’s fun to work in this way.” Oliver, Technical Application Lead, Gebrüder Dorfner GmbH & Co.
Capturing and Creating Knowledge
AI needs to be taught, both through experimental data, and through product experts imparting their domain expertise. This knowledge is used to focus the power of the AI model onto unknown areas rather than reinventing the wheel, speeding up development. Some AI platforms make extra efforts to be easy to use so as to capture the knowledge of the team using them.
Knowledge is captured as:
1. Data uploaded into the system rather than hanging around in a spreadsheet somewhere.
2. In an AI model itself as representation of what inputs affect what outputs.
3. In a search space, a description of constraints on formulations e.g. what ingredients can be used, what mixing parameters etc.
Expert knowledge and heuristics are encoded in AI models in such a way that it can be reused by more junior staff, now and in the future. An important consideration in an industry where many experienced formulators are about to retire.
As well as capturing the knowledge you already have in your team, AI can also uncover new insights. AI models work by figuring out connections between input and output properties.
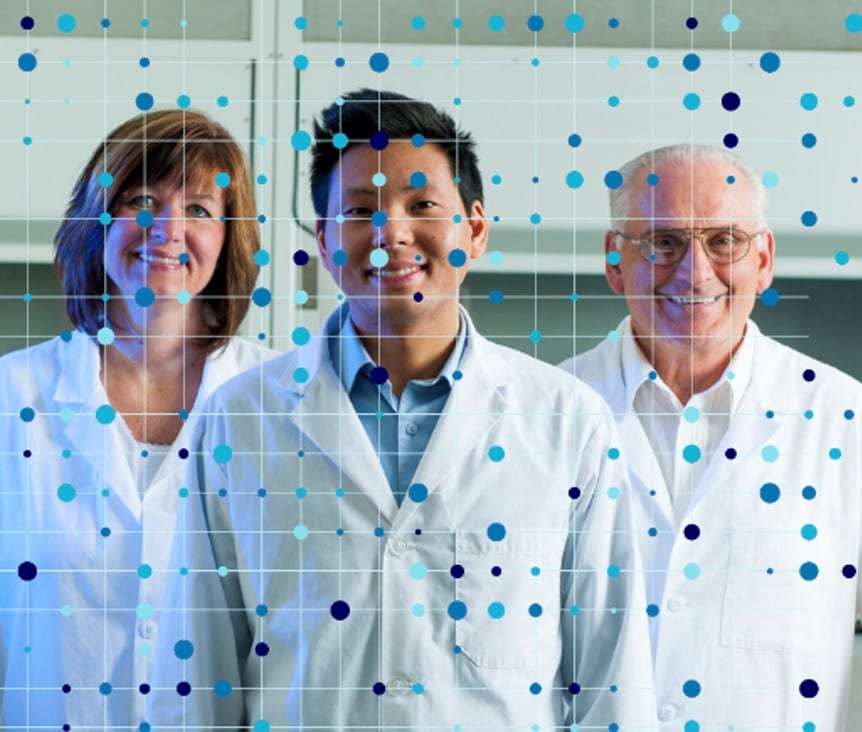
Some Companies Have Data in Silos
It may be that commercial information on ingredients is in an ERP system and peel strength measurements are in a LIMS. Or perhaps, your IP is stored in handwritten notebooks on a shelf? By running a short timescale AI project first, you will see exactly which data is useful for your AI model and therefore worth spending time digitizing. Any good AI provider will have an experienced team that can help you create a data strategy and get your relevant historical data into their platform. Data pipelines can be set up to ensure all future data goes in automatically.
What you need to look out for is whether the data model used by the AI provider is scalable. Can it easily accept data in different formats? As you learn that a different property is important in your project, can you easily add it? Or does it all have to go into one big rigid spreadsheet or SQL database? A graphical database structure such as the open source GEMD data model is ideal.
In summary, learn by doing. By starting with a small but valuable AI project, you will understand better which data you need and can put a data strategy in place that shows immediate value without boiling the ocean. Don’t get distracted by creating a data lake before you get value from AI.
But My Data Is a Mess…
You might need less data than you think. There is a difference between big-data AI, like ChatGPT and small-data AI. When it costs $100s or $1000s to create a sample and test it, datasets will always be small. Some AI companies have therefore spent the last 10 years focusing specifically on developing AI that works on the small datasets in materials and chemicals.
They do this by:
- Using the laws of physics and chemistry that determine the interaction between raw materials. These rules can be coded directly into AI models improving their accuracy.
- Focusing the power of the AI on a feasible search space by enabling experts to rule out experimental directions that they know won’t work.
- Chemical featurization (automatically generating extra data from chemical formula etc.).
- Uncertainty quantification (clever math to calculate the likelihood of hitting targets) to make best use of small data.
Some AI Projects Start with No Data
Sometimes, either by necessity or choice, projects start before any data in the relevant area has been gathered. In this case, an initial set of experiments is carried out, similar to a Design of Experiment (DOE) matrix but stripped down to cover the search space in the fewest experiments possible. The aim is to prime the AI model so that it can guide future experiments. Sequential Learning (the process by which groups of five or so experiments are suggested, run, the results inputted, and the AI model retrained and used to suggest the next set of experiments) is then used to get closer and closer to the objectives of the project. This methodology still requires fewer experiments than trial and error or DOE.
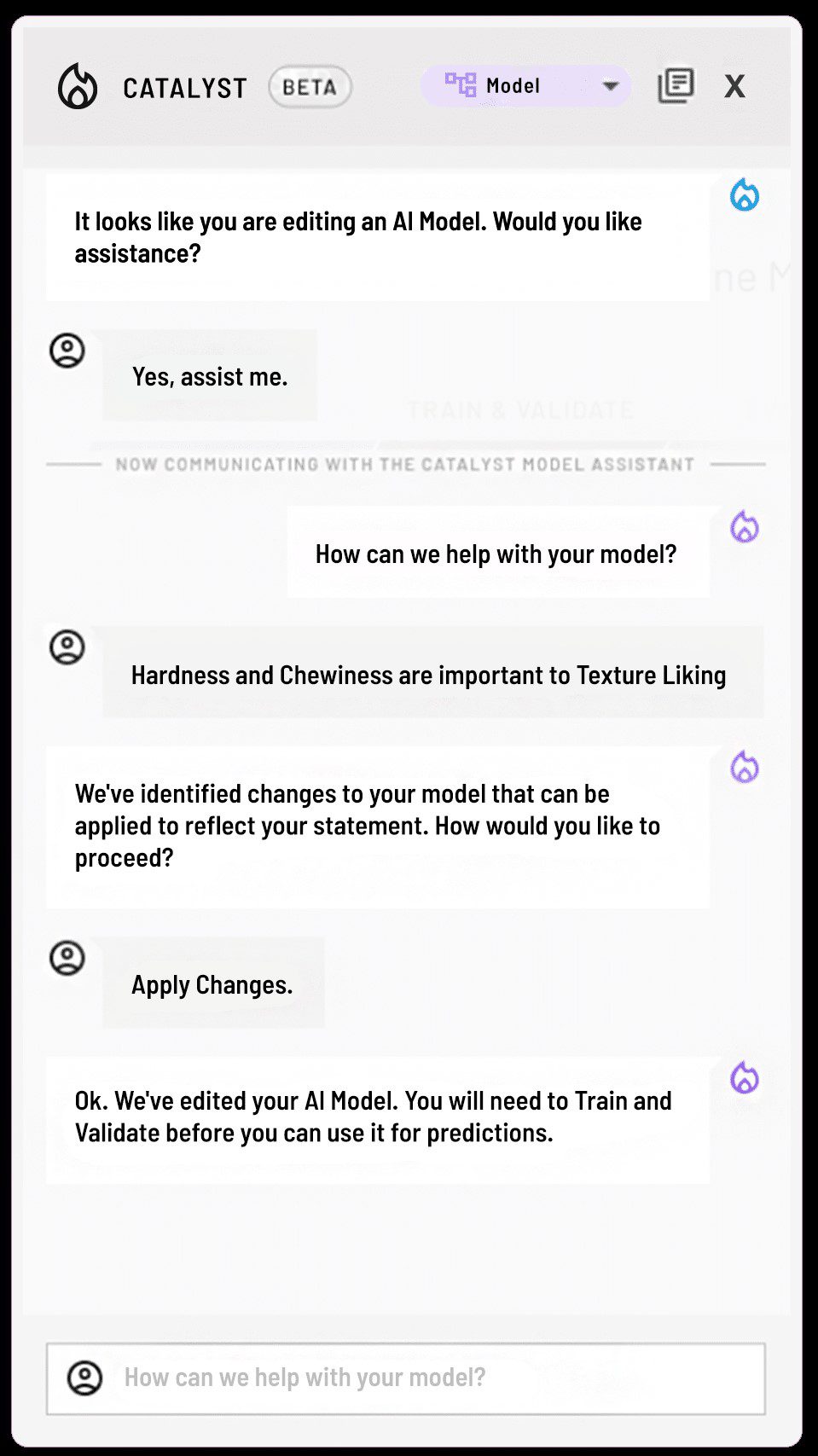
But I Don’t Have Any Data Scientists…
AI platforms have come a long way in the last five years. While they were once the domain of data scientists, the best ones can now be used by anyone. In fact, you want your formulators using them directly because they can then easily add their own knowledge into the platform to speed up development and also learn what the AI model is finding important.
No-Code, Graphical User Interface is Essential
While most AI platforms will have an API (code-based interface) that data scientists can use if they want to, making the graphical user interface intuitive and enabling formulation experts to add information such as relationships and custom formulas directly to AI models is important for both adoption of the new way of working and acceleration of progress. In some platforms, AI models are generated automatically from the data set chosen using some assumptions. A formulator must then just add their knowledge and sense-check the model. Some platforms even enable you to chat with the platform to refine the model.
Change Management
Adopting AI is a change to people’s day-to-day working styles and as such, presents a challenge. However, it is a small part of an overall digital transformation for many companies and an excellent way to show the value of good data management. By doing high-value, small AI projects first, you can show your team the value of their data and motivate them to participate in wider data digitization programs.
Some companies decide to make an AI platform freely available to the whole team after they have validated its usefulness on a few projects. But you shouldn’t neglect the continued need for change management until using AI is the new normal for the whole team. It’s a little like a bowl of fruit in an office. Everyone knows it would be healthy to go and get a piece of fruit, but they feel too busy to go and get it. They need a little extra support and encouragement to take an action that would benefit them in the longer term.
Don’t Expect Magic
Don’t expect AI to be able to instantly predict the properties you are interested in with 100% accuracy. Instead, treat it as you would a rookie formulator. Train it up with more data over time, showing it different scenarios that it hasn’t seen before, good outcomes and bad. It will learn faster than your junior formulator, and once trained it will be able to help the whole team.
What to Look for in an AI Provider
- Deep experience in AI for adhesives and sealants
- An experienced change-management team that can smooth the adoption process
- A platform that is chemically aware and provides chemical featurization
- A graphical data model that can accept data from lots of different sources and is scalable
- A no-code, easy-to-use platform that can capture your team’s expert knowledge
- Easy ways to search, filter, visualize, and share data between team members so that no one reinvents the wheel
- AI tailored to work with small data sets. Does it have great uncertainty prediction?
AI is inevitable. It is a great step forward in all sorts of disciplines. In the end, it is just math. Pick an easy-to-use platform backed up by an expert team to help you get started.
Learn more about Citrine Informatics at citrine.io.
Opening image courtesy of da-kuk / E+ / Getty Images.